Anomaly Detection Is Set to Revamp Manufacturing: Key Benefits and Use Cases
The applications of AI and ML in this sector are numerous, with anomaly detection being the most widespread and efficient. As experts in all things innovation, we will cover possible ML-based anomaly detection solutions and explain what business benefits they provide to manufacturers.
Anomaly Detection: Under the Hood
Before we explain exactly how anomaly detection solutions are able to enhance manufacturing processes, let’s start with the basics. ML is perfectly apt for anomaly detection due to its learning nature. Namely, machine learning runs its algorithms on specific datasets to find certain outputs — in our case deviations from the “normal behavior of a workflow.” Such training can be done using different methods:
- Supervised learning means leveraging labeled datasets in which anomalies are already marked. In this way, the ML model learns over time to distinguish between correct and incorrect outputs, i.e., normal and anomalous workflow patterns.
- Unsupervised learning occurs when ML models cluster unlabeled data, identifying hidden patterns by themselves. The process of sorting and grouping datasets happens by finding similarities and differences although categories are not provided. This can be data that does not fit well within any cluster or does not reconstruct well.
- Semi-supervised learning combines the first two approaches and is mostly used when only part of the input is labeled. The model trains on a few labeled samples and implements them iteratively to the rest of the unlabeled data.
Anomaly Detection Use Cases
By leveraging one of the learning methods to train your ML model, you can perform an array of complex tasks depending on your business case. Here are some of the examples of how anomaly detection mechanisms work in real life.
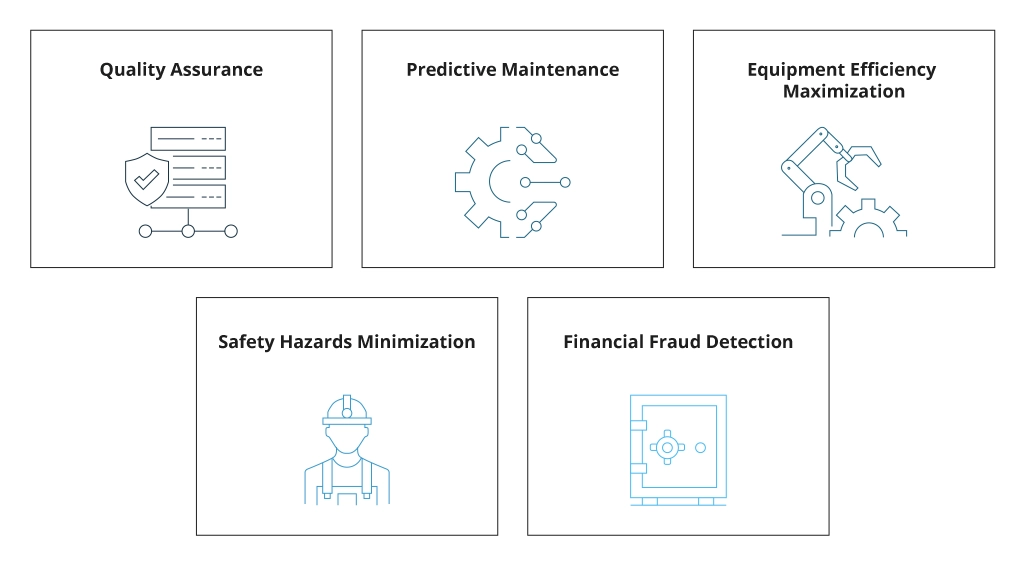
Quality Assurance
Zendesk states that 81% of customers are likely to repeat purchases after a positive customer service experience. Moreover, 70% say they make purchasing decisions based on customer service quality, and 64% of businesses report an increase in sales due to high customer satisfaction. These stats are proof that this is one of the key metrics for businesses, and in the case of manufacturing, high-quality production is what can make things work best once products enter the realm of customer service.
Before products go to customers, they should be verified for quality, including their structure and packaging. Human visual inspection is long and error-prone, and here is where automated anomaly detection comes into place. High-resolution cameras monitor each manufacturing stage in real time and the video footage generated during this process is further analyzed to identify all possible issues, including product defects, mislabeled items, and damaged packaging. Early anomaly detection paired with responsive problem-solving will eliminate the risk of delays in shipping and notably improve customer satisfaction thanks to high product quality.
A case in point: Infopulse set up a comprehensive analytics infrastructure for an international manufacturing company to enhance their production quality in casting stainless steel slabs. The solution helped slash a number of defects, reduce costs for defects detection, and boost manufacturing efficiency.
Predictive Maintenance
Downtime in manufacturing facilities is becoming more costly. According to a report by Siemens, incidents of downtime cost certain businesses up to $2 million an hour in 2022, and across all sectors that expense was at least 50% higher when compared to 2019-2020 data. The same study says predictive maintenance can save manufacturers 1.6 million hours of downtime annually and $734 billion through a 6% increase in productivity.
By predictive maintenance we mean using ML-based manufacturing anomaly detection to spot any deviations from the equipment’s normal performance long before the machine fails. Such a predictive maintenance solution integrates all relevant data from IoT sensors and smart cameras — to analyze equipment health, environmental data, machine specifications, and notes made by equipment operators. The insights are used to identify any shift from the normal performance, forecast impending failures, and/or perform early maintenance.
A case in point: Infopulse leveraged its machine learning and data analytics expertise to help a client detect anomalies in the operation of their gas turbines and also identify problem root causes. The delivered solution enabled 93% accuracy in anomaly detection, enhanced gas turbine monitoring efficiency, and reduced unplanned shutdowns.
Equipment Efficiency Maximization
Analyzing manufacturing equipment efficiency is key to identifying losses and areas of progress to further maximize productivity and profit. Anomaly detection can lend a helping hand here too.
By defining which devices stand idle for a prolonged period, manufacturers can take measures to balance load distribution, making all the operations run more effectively and minimizing equipment under-utilization. Conversely, by detecting the phases where the machine’s capacity is over-utilized, businesses can mitigate these occurrences and succeed in extending equipment life. The overall result is increased uptime and lower maintenance costs.
Safety Hazards Minimization
In 2020, the private manufacturing sector faced 373,300 nonfatal injuries (including illnesses) and 341 fatal cases. This resulted in 135,900 days away from work and 108,800 days of job restriction.
To avoid such alarming stats in your manufacturing settings, make the most of anomaly detection software. Fed with video footage from production facilities, the solution uses computer vision techniques like object detection and tracking, vehicle monitoring, and face recognition to spot employees who do not follow safety protocols and endanger their and their peers’ well-being. This might include not adhering to machinery maintenance rules, not wearing protection equipment, faulty actions, violent behavior, and more.
Another way you can define and quickly deal with dangerous behavior is by using wearables. IoT devices accurately collect data on employees’ health conditions and transfer it for ML-fueled analysis. The algorithms trigger alerts when workers are ill or overworked, and senior staff can encourage them to take a break or go on sick leave.
A case in point: Infopulse implemented our anomaly detection expertise to build an advanced mask wearing detection solution for our own office facilities. The delivered system offered 24/7 automated monitoring with up to 98% accuracy for mask detection and 95% for face recognition, which helped reinforce safety protocols within a matter of days.
Financial Fraud Detection
According to the 2022 report by the Association of Certified Fraud Examiners, an average organization loses 5% of its annual revenue due to fraud. For the manufacturing industry, that median loss is a stunning $177,000. With conventional methods in place, it is difficult to minimize losses, and here is where manufacturing anomaly detection eases the path to success.
Underpinned by ML, a robust 24/7 monitoring system can control all breach-prone operations in your organization. It is possible through identifying abnormal behavior, both in physical manufacturing settings and online, and flagging these situations for managerial attention. The use cases include the following:
- Log-on control uses normalized behavior modeling to spot anomalous patterns in accessing financial information by workers. An alert can be activated when a user tries to reach the data they have not used before, when the number of log-on attempts is exceeded, when the log-on location is rare, and more.
- Network intrusion detection means that ML algorithms analyze existing security data and behaviors as well as trends and connections to identify possible breaches and promptly react to them. Predictions help significantly reduce the time for malware detection, safeguarding your financial assets.
- Abnormal financial activity detection is another ML use case that can help manufacturers deal with fraud. Bogus insurance claims, money laundering, anomalous expenditures, suspicious transactions from different locations — the system spots anything that does not act in accordance with established norms and a worker’s role.
Anomaly Detection in Manufacturing: Key Benefits
Anomaly detection solutions can notably improve your manufacturing processes, and the above-mentioned cases are proof of it. Now let’s sum it all up and outline the benefits of implementing such an innovative approach.
Quality Control
The stats say that the automated industrial quality control market was $613.8 million in 2021 and by 2030 this number is projected to reach $1.02 billion, showing a CAGR of 5.8%.
Indeed, companies are investing more in automation in this respect because the value they receive far outweighs the costs to acquire it. In manufacturing, automated anomaly detection at every stage of your manufacturing process — from product preparation to its packing and delivery — means minimized shipping delays and higher end-user satisfaction.
Reduced Downtime
As we noted earlier, unplanned downtime costs companies not only a lot of money, but also reputation and time. And as opposed to conventional reactive measures, predictive maintenance can reinvent the whole process by reducing facility downtime by up to 15%, increasing labor productivity by almost 20%, and slashing new equipment costs by 5%.
Optimized Efficiency
All the use cases of machine learning anomaly detection mentioned earlier can contribute to enhancing overall productivity in the enterprise. This includes maximizing machinery efficiency through predictive maintenance and equipment underutilization detection, worker safety control, quality assurance, and other innovative approaches.
Cost Savings
The implementation of anomaly detection solutions may seem expensive during its early stages, but the value you get is certainly worth it. For example, predictive maintenance is highly cost-effective, saving manufacturers billions of dollars.
With the total incidence rate per 100 full-time employees being 3.1 in private manufacturing, investing money in workforce safety is also key to cost savings. Another way to reduce unexpected costs is by implementing automated financial fraud detection; as we highlighted above, this is particularly harmful for the manufacturing sector.
Conclusion
ML-based anomaly detection in manufacturing has proved to be effective for a number of parameters, and if you have not implemented this approach into your workflows yet, it is high time you did that — by partnering with an experienced developer like Infopulse. With multi-year experience in machine learning, computer vision, and business intelligence under our belt, we are ready to help with:
- Anomaly detection solution development and customization
- ML algorithms enhancement
- Manufacturing digitalization and automation consulting.
Send us an email describing your specific needs, and we will respond right away to schedule a consultation.