What Is Deepfake Detection in Banking and Its Role in Anti-Money Laundering?
Financial institutions are facing a new breed of criminals armed with the power of artificial intelligence. In 2023, the banking industry saw a massive increase in financial fraud using deepfakes ā hyper-realistic synthetic media. According to the Identity Fraud Report, there was a 3,000% rise in deepfake attempts to bypass security measures. This translates to a 31-fold year-over-year jump, highlighting the urgency of the situation.
The cause? The democratization of generative AI and user-friendly face-swap apps. These tools have empowered criminals to craft ever-more convincing deepfakes, jeopardizing the integrity of identity verification and opening a backdoor for sophisticated financial fraud. In 2023, nearly 60% of banks, fintechs, and credit unions experienced direct fraud losses exceeding $500K.
This alarming trend shows that financial institutions need to update their security measures beyond standard Know Your Customer (KYC) checks to tackle new digital threats.
Types of Financial Frauds Enabled by Deepfakes
The complex technology behind deepfakes allows them to generate hyper-realistic forgeries of video, audio, and other media formats. This is morphing them into a versatile weapon for financial criminals.
Hereās what stands behind deepfake technology:
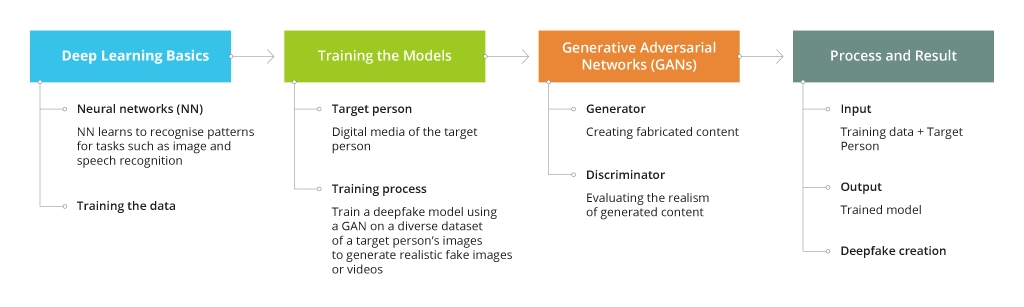
Let's take a glimpse into the different ways criminals are leveraging this technology:
- Identity theft. Deepfakes can impersonate legitimate customers during account opening or verification, thus bypassing standard checks. A Regula survey paints a concerning picture: 37% of organizations encountered deepfake voice fraud, and 29% have fallen victim to deepfake video scams. While AI-generated deepfakes are a growing concern, another cunning tactic is on the rise: synthetic identity fraud, also known as "Frankenstein IDs." Just like its namesake monster, these identities are stitched together from bits and pieces of real and fake information to create entirely fabricated personas. According to a Regula survey, nearly 46% of organizations globally faced this "Frankenstein" threat in 2022. Fraudsters use these synthetic identities to open fraudulent accounts, make unauthorized purchases, and disappear with the loot ā leaving financial institutions holding the empty bag.
- Social engineering scams. Deepfakes are used to manipulate bank employees or customers into authorizing fraudulent transactions. Hackers are employing advanced social engineering tactics, creating fake websites, deploying "digital tailgating or piggybacking " (exploiting the userās credentials or current session through unauthorized access), and even crafting chatbots and voice conversations that mimic real people. This, coupled with AI-powered algorithms, allows them to bypass traditional security measures. A prime example: the infamous MGM Casino hack, where deepfakes reportedly facilitated a system-wide outage and a hefty $100 million heist.
- Synthetic media fraud. Unlike traditional identity theft, which relies on stealing real people's information, synthetic media fraud cooks up entirely fictional personas. This makes it incredibly difficult to detect using conventional methods. Another Identity Fraud Report shows a 10x rise in deepfakes detected worldwide from 2022 to 2023. Crypto and fintech made up 96% of these cases. Deepfake incidents in fintech alone increased by 700% in 2023, highlighting the need to screen out malicious actors during verification.
- Fraudulent loan applications. Deepfakes can be used to create realistic identities with fabricated employment history, income verification, and even references. These fake personas can then be used to apply for loans or credit cards, which are then defaulted on. The cost of this forgery is staggering ā a total of $3.1 billion is at risk from suspected synthetic identities for U.S. auto loans, bank credit cards, retail credit cards, and unsecured personal loans, the highest level in history.
- Money laundering schemes. Deepfake technology is widely employed to create fake accounts or disguise the identities of individuals involved in money laundering activities. Criminals can use these fabricated personas to move illicit funds through the financial system undetected.
Additional Emerging Threats
- Impersonation during customer onboarding (video KYC). Deepfakes can bypass video-based KYC procedures. The Federal Trade Commission reported that impersonation scams cost victims about $1.1 billion in 2023.
- Manipulating video evidence of transactions. Though the use of deepfakes, hackers can alter video recordings of transactions to mask fraudulent activity. For example, after a group video conference call employees were instructed to send $25 million to scammers. Hackers usually try to fake liveness checks with a very simple trick: they send in a video of a video playing on a screen. Currently, more than 80% of attacks are based on this method.
- Spoofing voice calls for authorization. Criminals are leveraging deepfakes to mimic the voices of legitimate users to gain access to voice authentication systems and authorize unauthorized transactions. Here's how it works: Fraudsters can record a target's voice from intercepted phone calls, voicemails, or even social media videos. Using deepfake technology, they can manipulate these recordings to create a synthetic voice that closely resembles the target's one. This synthetic voice can then be used to fool voice authentication systems, granting access to accounts or authorizing financial transactions.
Challenges in Deepfake Detection for Anti-Money Laundering
- Data limitations. Training effective deepfake detection models requires a vast amount of real-world data, both genuine and manipulated. Banks may struggle to access or share such sensitive data due to privacy concerns. AML systems may not have the necessary volume or variety of data to train robust deepfake detectors.
- Evolving technology. Deepfake creators constantly improve techniques, making detection a moving target. Criminals can adapt their techniques to evade current detection methods. This continuous evolution necessitates AML systems to stay updated with the latest deepfake creation methods.
- Explainability and transparency. ML models can be complex, making it challenging to understand how they arrive at a "deepfake" conclusion. Regulatory bodies might require transparency in how these models flag suspicious activity to ensure they are not biased or prone to errors. Without clear explanations, AML specialists might struggle to justify actions taken based on the model's output.
Deepfake Detection Techniques for Banks and Financial Organizations
To fight fraud, 75% of banks and fintechs plan to invest in an identity risk solution.
This end-to-end platform manages identity, fraud, credit, and compliance risks throughout the customer lifecycle. Letās take a closer look at some other deepfake detection techniques employed by banks within their anti-money laundering systems.
Process Review
First, conduct a detailed review of all your processes to understand how threats are detected and addressed. A well-built process can mitigate the majority of attacks itself, by design. You may not even need AI-powered threat mitigation solutions to detect most attacks. Because if you have such simple technologies as an online risk verification solution, (e.g., Identity protection solution for M365/O365), MFAs, OTPs and many more, you will be able to block 99% of all the attacks initially. While more advanced threats will be further handled by AI-powered solutions.
Multi-modal Analysis
The multi-modal approach combines different detection methods and simultaneously analyzes facial features, voice patterns, and user behavior during interactions to identify inconsistencies. In fact, by 2026, 30% of enterprises will stop relying solely on face biometrics for identity verification and authentication due to AI-generated deepfake attacks, according to Gartner. Moreover, if comparing face biometrics with a classy OTP (one-time-pass) over plaintext SMS, the latter would be a safer approach to verify a personās credentials.
AI-powered Detection
AI deepfake detector market is expected to hit USD $5.7 million by 2034. Machine learning algorithms trained on massive datasets of real and manipulated videos can identify subtle artifacts left behind during the deepfake creation process. For instance, AI can compare lip movements in a video with the corresponding audio track to detect any mismatch that might indicate a deepfake. Additionally, this approach can analyze the texture and lighting of images, the cadence and pitch of voices, and even the context of the communication to cross-verify the authenticity of the presented identity. This allows banks to ensure robust security measures in their AML systems, significantly reducing the risk of fraud.
Alternatively, you can use hardware tokens or even offline one-time passwords (printed ones) in highly secured environments. Such tokens or OTPs can be handed to specific people related to your organization or they can be simply generated in a protected app. Combined with other layers of security, they donāt rely on any attack vectors that a deepfake technology could exploit.
Biometric Authentication
Unlike a face in a video or a voice recording, biometrics is much harder to replicate convincingly. For example, fingerprint scanners measure the unique ridges and valleys on a user's fingertip. Replicating these intricate details with enough accuracy to fool a scanner is extremely difficult. Similarly, iris scans analyze the intricate patterns of the colored part of the eye, providing another layer of security.
While facial recognition can also be a form of biometric authentication, deepfake advancements (especially high-quality deepfakes) undermined all possible facial recognition systems, prompting ongoing debates about user privacy and potential biases within facial recognition algorithms.
Discover more about Physical and Behavioral Biometrics in a related article.
Continuous Behavioral Monitoring
Banks are increasingly incorporating behavioral analytics into their AML systems. Machine learning solutions analyze a user's historical data, including transaction patterns, login locations, and device types. Together with online risk verification, MFA, OTPs and other tried and tested technologies, you can establish continuous behavioral monitoring to raise red flags:
- Transaction pattern analysis: Machine learning algorithms can analyze a user's historical transaction data, including average transaction amounts, frequency, and location. A sudden surge in spending, significant transfers to unfamiliar accounts, or transactions originating from unusual geographic locations could all trigger an alert, especially if they coincide with a suspected deepfake attempt during KYC verification.
- Login location tracking: Banks can monitor a user's typical login locations and devices. If a login attempt originates from a geographically distant location or an unfamiliar device, particularly during critical actions like video KYC or high-value transfers, it can raise suspicion. This can be particularly useful in identifying attempts to impersonate a user through social engineering tactics combined with deepfakes.
- Device identification and analysis: the type of device used for login attempts can also be a valuable data point. If a user typically logs in from a laptop but suddenly attempts video KYC from a mobile device they've never used before, it could initiate further investigation.
Conclusion
The battle against deepfake fraud requires a layered approach. Banks need to combine technological advancements with human expertise and risk-based assessments. Continuous research and development in deepfake detection offer promising solutions, such as advanced liveness detection for video KYC and voice authorization systems. As the fight against deepfakes evolves, so will the AML systems be improved to protect the financial security. Infopulse stands beside financial institutions in this strategic game, providing the tools and expertise needed to stay several moves ahead of the evolving deepfake threat.