Hyperautomation in Agriculture and Precision Farming: How to Optimize Complex Tasks
Agriculture has recently faced a number of challenges, with inefficient resource usage and weather variability being key among them. As a response to this problem, precision farming appeared and started gaining traction. This is a concept that uses hyperautomation technologies, including AI, ML, IoT, computer vision, and business intelligence, to enable sustainable agriculture practices.
According to Precedence Research, the worldwide precision farming market was $9.8 billion in 2022, and this number is expected to reach about $34.01 billion by 2032. The level of growth is not a surprise considering the value agronomists receive.
As an IT services provider with mature intelligent automation services, we constantly monitor market trends. In this article, we’ll cover how agribusiness can optimize agriculture and precision farming tasks using hyperautomation.
Learn more about precision farming and its impact on agribusiness development!
Precision Agriculture: Data Lifecycle Management
Comprehensive data collection and analytics are required in order for farmers to make smart decisions on irrigation, crop management, fertilizer use, and other complex agricultural tasks.
Data Collection
To get valuable data on lands and crops, precision farming implements advanced IoT technologies:
- IoT sensors are smart gadgets that are affixed to soil, plants, or drones to understand chemical or physical changes in the environment and transform them into electrical signals for further processing. Examples include optical sensors for light prevalence measurement, electrochemical sensors to process chemical soil data, mechanical sensors for soil compression analysis, dielectric sensors to gauge moisture levels, and more
- Unmanned aerial vehicles (UAVs), or drones, are aircraft that leverage sensors and cameras to capture very high resolution (VHR) images and videos of fields and plants. Such aerial data is further used for digital image processing, mapping, and photogrammetry
- Smart cameras installed on tractors gather information on fields and crops in the form of video footage. Then, computer vision tools are introduced for deep analysis
Hyperautomation technologies accelerate the whole process of data collection and entry for both agriculture-specific and back-office operations. Workflow automation here results in enhanced efficiency, faster task execution, and significant time saved for human workers.
Data Aggregation
The data collected from numerous connected devices is usually unstructured and varies in source and type. To be able to derive meaningful insights from it, primary preprocessing is needed. This can be done with advanced AI algorithms that automatically incorporate and consolidate data from multiple sources, perform validation and massaging, i.e., eliminate inconsistencies and resolve conflicts. The next step is to convert data into a specific, more digestible format through sorting, grouping, and also using AI-powered techniques.
All these processes happen in a cloud-based warehouse that acts as a unified data source, enabling data integration and collaboration across different systems. At this phase, you will also need GIS tools and sophisticated ML algorithms to present data in an intuitive, map-based format and be able to scrape data and make updates when needed.
Data Analysis and Visualization
When data is collected from smart sensors and other IoT gadgets, integrated into a single warehouse, and processed by AI, in-depth analysis comes into play. An advanced data analytics platform underpinned by ML helps you analyze huge data volumes by finding correlations and hidden patterns between variables. Automated data cleaning and feeding into ML algorithms also happen at this stage. Algorithms handle missing values and outliers, eliminating inconsistencies. The result of such an analysis includes insights into plants’ humidity, shape, temperature, chemical levels, size, etc. — that are further used to improve crop monitoring, land management, chemical usage, irrigation, and more.
The advantage of using an analytics platform here is that all the insights are presented in interactive data analytics dashboards that are easy to use and update. Also, you can visualize your agricultural data in custom reports for better decision-making.
Specific Hyperautomation Use Cases
Now that we have explained how data processing and analysis takes place in hyperautomation workflows, let’s delve into the examples of how you can use the power of tech to get a competitive edge in agriculture.
Hyperautomation Techs in Agriculture: Use Cases
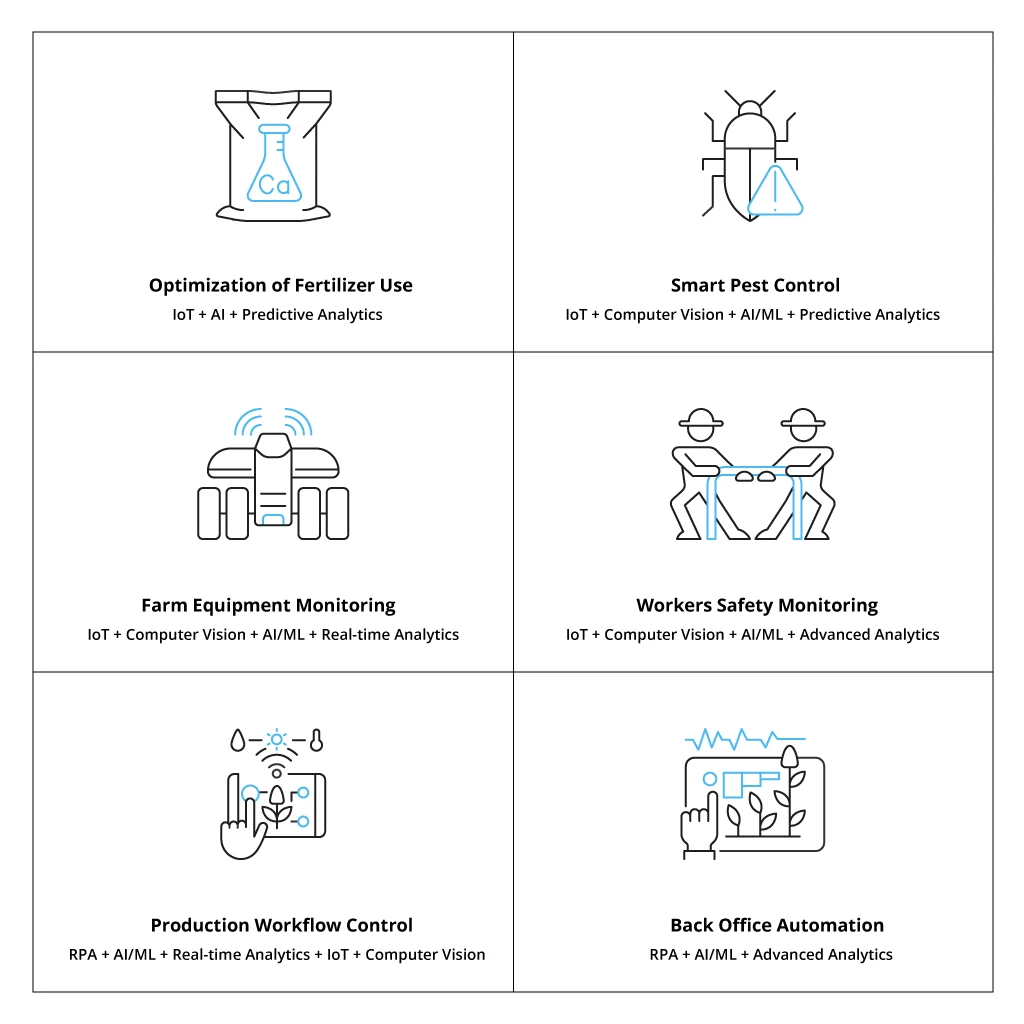
Fertilizers Use Optimization
The latest stats show that farmers are struggling right now. Rising input costs are their leading risk to overall profitability, with the high price of chemicals and fertilizers at the top of that expenditure list. On top of that, the situation might be aggravated for years to come, and here is where intelligent fertilization planning can help.
Numerous benefits arise by leveraging AI-powered sensors and implementing a sophisticated analytics system into your farming processes. Sensors installed in a raised bed garden are responsible for measuring the status of your soil’s nutrients; namely, properties like soil pH, salinity level, temperature, and moisture. The collected data is then transferred to a cloud-based analytics platform driven by AI algorithms to gauge how much input from the last year is available for this year’s crops and predict the development and yield of future crops considering weather conditions.
The data will also help analyze how the fertilizer impacts the growth of a crop and define its exact amount. The knowledge about the soil nutrient levels helps notably improve fertilization schedules and avoid using an excessive amount of fertilizers — which results in reduced input costs and better environmental sustainability.
Smart Pest Control
Up to 40% of annual worldwide crop production is lost due to pests, which costs the global economy about $70 billion. To eliminate this challenge to efficient food production, farmers can leverage hyperautomation. This can be done by different methods:
- Low-power sensors and cameras installed in connected traps instantly detect the insects and send the captured images to a monitoring platform. Then, computer vision is introduced to recognize the pests, identify their number in each trap, and spot their activity patterns. To analyze those patterns against the historical data, machine learning algorithms can be used. With this, farmers can identify the exact locations of insect infestation and take measures toward eliminating them.
- Thermal and infrared sensors measure the spectral signature of plants and soil to promptly detect pest attacks. Once a pathogen covers a plant’s leaves, an alert is sent to a central monitoring platform using AI/ML, anomaly detection, and predictive analytics.
- Fluorescent sensors powered by optical cameras help signify changes in a crop’s chlorophyll patterns, analyzed by AI to detect pests or pathogens.
- Acoustic sensors are able to pick up pest sound waves, indicating locations with high concentration of insects.
The implementation of such sensors, together with AI/ML and intelligent analytics platforms, will help to not only improve crop health, but also optimize the use of pesticides.
Worker Safety Monitoring
According to a report by the Bureau of Labor Statistics, agriculture is one of the most hazardous occupations in the world, with an incident rate reaching 4.6% in the US alone. This includes nearly 500 fatalities for the years 2019-2021. Such alarming stats are a huge impetus for implementing automated worker safety monitoring on the farms.
Farmers’ and agricultural employees’ health can be analyzed in real-time by AI/ML algorithms coupled with advanced analytics through the information collected by sensors and cameras. Heart rate, blood pressure, glucose level — by monitoring such key aspects, you can quickly and accurately detect negative changes in health conditions and in turn prevent accidents.
IoT gadgets (aka wearables) are also helpful in constantly monitoring a worker’s position and status in remote locations and potentially hazardous scenarios; for example, when working with heavy machinery or large animals. If an emergency happens, the farmer or worker can activate a specially designed Bluetooth panic button. They can also communicate with the team through 10- or 15-minute check-in timers. Once that person misses such a check-in, coworkers will be automatically notified and emergency help will be sent to their location.
Farm Equipment Monitoring
Expenditures on agricultural machinery are quite high. In the US alone, agronomists spent $19.1 billion on self-propelled farm machinery and tractors in 2022. On top of that, the restrictions imposed by machinery manufacturers might cost farmers weeks or months of downtime, which translates to $3 billion a year.
To avoid such costly downtime that inevitably leads to a suboptimal harvest, you can implement real-time machinery monitoring and real-time analytics using sensor technology and smart cameras. A deep ML-based analysis of the data collected by these IoT tools will help you constantly check machinery health and detect any malfunction in its early stages. You can set specific threshold limits and tolerances around machinery performance. Once surpassed, an immediate alert is activated so that you can prevent possible breakdowns.
Computer vision mechanisms are also key to ensuring machinery safety. They can be programmed to detect improper worker behaviors and equipment misplacement — to alert the team to take the necessary measures. Even if an accident happens, an advanced computer vision-based system will help unveil and analyze the root cause to eliminate the risk of similar incidents happening in the future.
Production Workflow Control
Behind the very process of sowing and harvesting agricultural products, there is an array of other key operations that can be hyper-automated to take your smart farm to the next level:
- Inventory management automation means you always have up-to-date information on every product in stock, from fertilizers and seeds to machinery and fuel. Once the stock reaches low values, you are instantly notified by AI chatbots coupled with RPA to automatically gather data on the inventory levels. Control of the essentials helps you sow, irrigate, fertilize, and harvest on time. You can also enable accurate, real-time demand forecasts if coupled with cameras and IoT sensors and mitigate supply chain disruption.
- Supply chain management presupposes leveraging AI and other hyperautomation tech to analyze vehicle capacities, transportation routes, delivery schedules, and other variables to optimize the transfer of farm products to the market. In this way, agronomists can not only minimize delivery time for improved customer satisfaction, but also reduce transportation costs and waste.
- Quality control is about using computer vision and ML to analyze the quality of agricultural products. By taking into account specific parameters such as product size, color, and form, automation mechanisms can identify anomalies and defects — both in the very products and in the way they are packed before being transferred to the customer.
Read more about Intelligent Defect Inspection to Optimize Monitoring using Computer Vision and Deep Learning
Back Office Automation
The efficiency of performing back-office workflows directly influences the overall business success, no matter the industry. That is why the demand for back-office automation has grown recently. According to The Insight Partners, the back office workforce management market was $3.60 billion in 2022, and is expected to reach $6.71 billion by 2028.
Agriculture is no exception. By implementing RPA, AI, and advanced analytics into an enterprise resource planning (ERP) and/or customer relationship management (CRM) system, you can automate the following tasks:
- Finance management can be supported by RPA bots that gather necessary data, transfer it to AI that analyzes intel through the advanced analytics system, which further generates insights to empower farmers with visibility into all the key operations around accounting, procurement, cash flow, payroll control, and supply chain management. By controlling all this data, you can easily forecast field profitability and break-evens — to wisely plan seasonal budgets.
- Market analysis makes the best of AI to analyze real-time and historical data on product prices, consumer behavior, and competitors to improve strategic decision-making and be able to proactively respond to market fluctuations.
A case in point: Infopulse enhanced an agricultural CRM solution with advanced analytics capabilities to help Europe's leading crop breeder and seed producer notably improve production planning, product quality control, and sales activities.
Conclusion
Smart farming is an overarching concept that brings together an array of technologies and complicated business tasks that can be hyperautomated. As experts in innovation, Infopulse can help you with any task throughout the precision agriculture operations lifecycle:
● Business intelligence platform development and customization
● Enterprise-grade IoT software development
● Enterprise software enhancement
Drop us a line with your business query, and we will get back to you to discuss your particular case.