Challenges of Big Data Analytics to Consider Before Implementation
Quick and accurate data analytics is essential in getting to know the market and customers better, discovering new possibilities for growth, and making evidence-based decisions to boost profits.
Enterprises are experiencing the drastic increase in data volumes – the amount of total enterprise data summitted from 1 petabyte in 2020 to 2.02 petabytes in 2022, which means 42.2% average annual growth over two years.
This and the inability to process such volumes of data greatly accelerates implementation of big data storages and analytics tools. Yet, enterprises face numerous challenges of big data analytics while integrating such tools into their digital infrastructures and data workflows.
In this article, you will discover how to implement big data analytics and derive smart insights from all types of data, minimizing failure and financial loss risks.
How Big Data Analytics Helps Business?
Big data gains strategic importance across numerous industry sectors, helping enterprises handle their ever-growing data and transforming it into a profit-generating resource. A recent survey shows that 39.7% of companies already manage data as a business asset.
Here are a few use cases of big data analytics in different industries:
- E-commerce: revealing trends in customer behavior and demands, extending the assortment of products/services based on customer expectations, forecasting pricing and stock.
- Marketing: driving high ROI marketing campaigns to improve sales and conquer new customer segments as a result. See how Infopulse implemented a market data analytics solution for Gorenje Group.
- Banking and Finance: optimizing business processes, extracting customer income and spending patterns to forecast the likelihood of choosing certain banking offers and services (loans, credit cards, deposit accounts, etc.), fraud detection.
- Telecom: forecasting network capacity and improving customer experience. For instance, Infopulse implemented a modern Big Data Platform solution for BICS, a world-class telecom services provider, to accelerate the processing of massive signal data and enable real-time analytics for decision-making based on the latest information available. As a result, the company got twice faster data analytics within 1.5 traffic increase.
- Healthcare: analyzing patients' medical history helps predict the risks of health issues.
- Education: developing new courses and specialties and improving existing educational programs based on the latest market demands.
- Media and entertainment: analyzing statistics to understand the demand for specific types of shows, movies, songs, and other creative content. Big data analytics is also used for personalizing recommendations and offers to customers. Here’s how a German media content provider improved its customer service with powerful personalized recommendations and reduced the data infrastructure costs with an ML-based big data analytics solution developed by Infopulse engineers.
- Manufacturing: supply chain management, resource management, and operations control along with IoT connectivity technologies. A resource management application developed by Infopulse helped the client streamline the resource management across 100,000 employees worldwide and enable accurate unified reporting from multiple ERP systems.
- Government: with more strategic insights at hand, authorities will be able to take data-driven decisions in law enforcement.
If you would like to know more about AI and big data and how they disrupt the manufacturing industry, click the link to our dedicated article.
There can be different objectives for investing in big data analytics solutions. Besides delivering higher quality customer experience and customer retention, it helps reduce the response time to market changes and reduce the time to market for new products and services. Big data analysis helps in gaining a competitive advantage in the market. Besides this, it can also be used to optimize the internal processes in the company, as it reveals ineffective processes and reduces wasted costs by streamlining resource-consuming processes.
Benefits of Big Data Analytics for Business
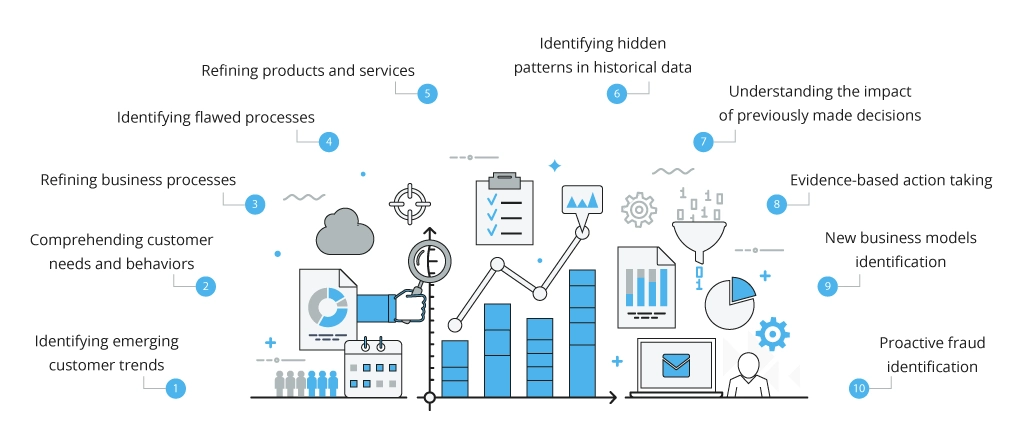
Potential Challenges of Implementing Big Data Analytics
Though the market size of global big data and analytics services is projected to grow from $121.65 billion in 2022 to $221.44 billion in 2027 at a CAGR of 12.7%. Some big blue-chip enterprises are still struggling to maximize their business potential from big data environments. Adopting big data analytics poses specific challenges that should be considered when the organization decides to implement it.
Data Resources and Management
An organization that plans to implement big data analytics system should assess the computing system and data storage capacity not only to contain all the data but also to perform the analytics and visualization. Investing in the right data architecture to accommodate the planned big data volumes and processing capabilities is crucial before designing and implementing big data analytics solutions.
Security and Compliance
Integrating big data analytics requires additional security tools and operations, including:
- Securing non-relational data with hashing or encrypting passwords;
- Strengthening internal security via proper access control, authorization mechanisms, and real-time data security monitoring;
- Setting up a reliable data storage with a secure untrusted data repository (SUNDR) to detect any unauthorized changes in data files;
- Ensuring endpoint security with trusted certificates.
Among others, you should also account for industry-specific regulations, governmental regulations, and high security standards:
- PCI DSS: This security standard applies to all organizations that collect, store, process, or pass customers’ payment data.
- HIPAA: All healthcare organizations and associated businesses adhere to HIPAA requirements that protect patient information confidentiality and regulate the terms of its use.
- GDPR: This uniform data security law protects EU customer data. Any businesses in any country that deals with EU residents must comply with the extensive requirements of the GDPR.
Skills and Expertise
The recent report from EY revealed that 53% of senior executives consider data and analytics technologies as the top area of investment for the next two years. However, 19% of executives lack the relevant knowhow to execute their data-centric strategies.
Proficient big data skills and technical expertise are one of the biggest challenges in implementing big data analytics for enterprises. Normally, companies feel great shortage or even absence of data scientists and experts in related areas. Recruiting such talents also poses additional expenses for the organization. For that reason, many enterprises decide to outsource their big data analytics efforts to dedicated service providers.
Resource and Budgeting
Resource considerations include not only the cost of the analytics system. The budget for the implementation of big data analytics budget also includes the price for hired specialists, staff training and onboarding, and post-deployment system support and maintenance. You should always keep in mind that collecting and analyzing data with advanced tools requires significant investments that are worthwhile only if they bring tangible results.
Data-Driven Decision Making
Understanding the outcomes your organization gets from adopting big data analytics will help you clearly define the key features your solution must contain. If you want it to solve specific business tasks, then opt for a strategic approach for successful design and implementation. Discover how we helped a global audit company accelerate its decision-making with big data analytics.
How to Build a Strategy for Implementing Big Data Analytics?
Most potential challenges of big data analytics can be effectively addressed by designing a strategy for adopting big data analytics. Often companies need a comprehensive view of all their data stored in disparate departmental systems that lack integration, consistency, format unity, and visibility. In addition, the quality and trustworthiness of data sources become dubious. Infopulse helped a global audit company, One of the Big 4, to address these challenges by implementing a custom Big Data application on AWS. The solution consolidated data from numerous disparate sources without duplications and other inaccuracies. With robust big data analytics, the solution brought the company’s services to a new level.
Inability to evaluate the available resources and define the desired project goals doesn’t allow companies to build a well-thought-out data analytics strategy. As a result, they jump into the development phase with no detailed roadmap. However, without a carefully planned strategy in place, enterprises end up with solutions that do not align with their objectives or require significantly bigger investments to adjust the system to operate adequately on the go. Hence, much effort is wasted trying out different solutions to find the suitable one. In addition, all that has a drastic impact on ROI for big data analytics.
Therefore, you should build a strategy that primarily meets your long-term strategic objectives, avoiding duplicate or competing efforts, while keeping in mind expenses optimization.
The strategy design includes four steps:
Step 1. Define business goals and objectives
Big data analytics itself is a tool for achieving specific results. You must clearly understand what you need it for and what results it should bring to add business value. Involve stakeholders from business leaders, the data management team, data engineers, and other departments that deal with data analytics.
Step 2. Identify Data Sources and Processes
Define the variety of big data sources, assets, and business processes related to data analytics, and evaluate your current technology resources and capabilities. Match your findings with your business cases and objectives to define the gaps in the existing infrastructure and other issues. The assessment of the current state of IT assets will help determine available and missing resources for implementing the future solution.
Step 3. Identify and Prioritize the Use Cases for Big Data Analytics
Define the use cases that meet your business objectives from the first step. Understand and document the exact patterns, correlations, and other insights you need to extract with the help of your analytics system. Then prioritize all use cases based on business impact, required resources, and expenses. Based on prioritization, finalize the scope of use cases to cover the future solution.
Step 4. Build the Project’s Roadmap
A roadmap is an outline of the future big data analytics implementation. It can be created in iterations, starting from basic project structure, work scope definition, and development stages timeline. It is critical to have a shared vision and roadmap for all enterprise branches and departments, to align all individual initiatives and needs with the overall strategic priorities.
Based on the defined goals, current state, and business case analysis, propose a solution for implementation. Stakeholders, data scientists, software architects, and developers should work together to identify a solution that addresses all the outlined business needs. Considering the mix of team skills, data governance frameworks, and data processing, a delivery model should be proposed within the roadmap.
How to Design a Roadmap for Implementing Big Data Analytics
An effective roadmap for an organization is based on the current state of its data and analytics capabilities. It defines the desired outcomes from the future solution and provides a plan for achieving them. It helps avoid the multiple challenges of big data analytics implementation, interpreting the strategy into a clear to-do plan for the software development team. Here is how to develop such a roadmap.
Feasibility Study
This step includes validating the practicality of the future solution, calculating the budget for implementation and ROI, and estimating the operational costs.
Engineering Technical Requirements and Big Data Solution Planning
In this step, the project team defines all data types to be gathered and stored and estimates data volumes and quality metrics. It also involves formulating a high-level vision of the future solution, including:
- Required data storage capabilities
- Integrations with existing components of the IT infrastructure
- Data processing specifics
- Security and compliance requirements
- Analytics processes and results
- Deployment model
- Technology stack
The range of the solution components depends on the individual specifics of each project. The final result of this step is a detailed project plan with timeframes, a required team set, and outlined budget.
Architecture Design
The architecture design consists of the following:
- Data model creation
- Outlining data security and data quality management mechanisms, including access control, data encryption, redundancy, and other approaches.
- Big data architecture design for data ingestion, processing, storing, and analytics.
Within technical requirements, architecture design, and the project implementation plan, you can move to big data solution development.
Conclusion
Big data tools bring businesses the powerful potential to understand their customers better and forecast future market development. Constantly growing amounts of data make its processing and analysis more challenging, driving the active adoption of big data analytics.
To implement a practical solution, you should consider all the potential challenges of big data analytics beforehand, assess the current state of your data infrastructure and technical and resource capabilities for improvements, and match them with your expectations and business goals. Based on that, formulate the big data strategy and the roadmap for future solution development.
Infopulse data engineers and software development experts help clients from various industries generate smart insights from any type of raw data to make data-driven, intelligent business decisions. We help enterprises implement effective analytics solutions using the latest big data processing and storage tools, including Azure Analysis Services, Microsoft Power BI, Azure Synapse Analytics, SAP Analytics Cloud, and many others.